Learning in Control
Algorithms of artificial intelligence and machine learning are of increasing importance for control applications. Our research and expertise in this domain ranges from the modeling of unknown or uncertain dynamics over iterative and reinforcement learning to Bayesian optimization.
One research focus at the Chair is on learning in model design and identification. Hybrid and data-driven models are attractive if physical modeling is either poor or requires high effort. Practical applications often require an online adaptation of these models in order to reflect effects of aging or wear or to increase the model accuracy in different operation regimes. Information about the reliability and trustworthiness of a learned model can directly be used within the control design. For instance, the prediction of the uncertainty allows to satisfy constraints with a given probability. A challenge with learning-based methods is to ensure real-time feasibility with possibly weak hardware resources in order to bring these advanced learning in control methods into practice.
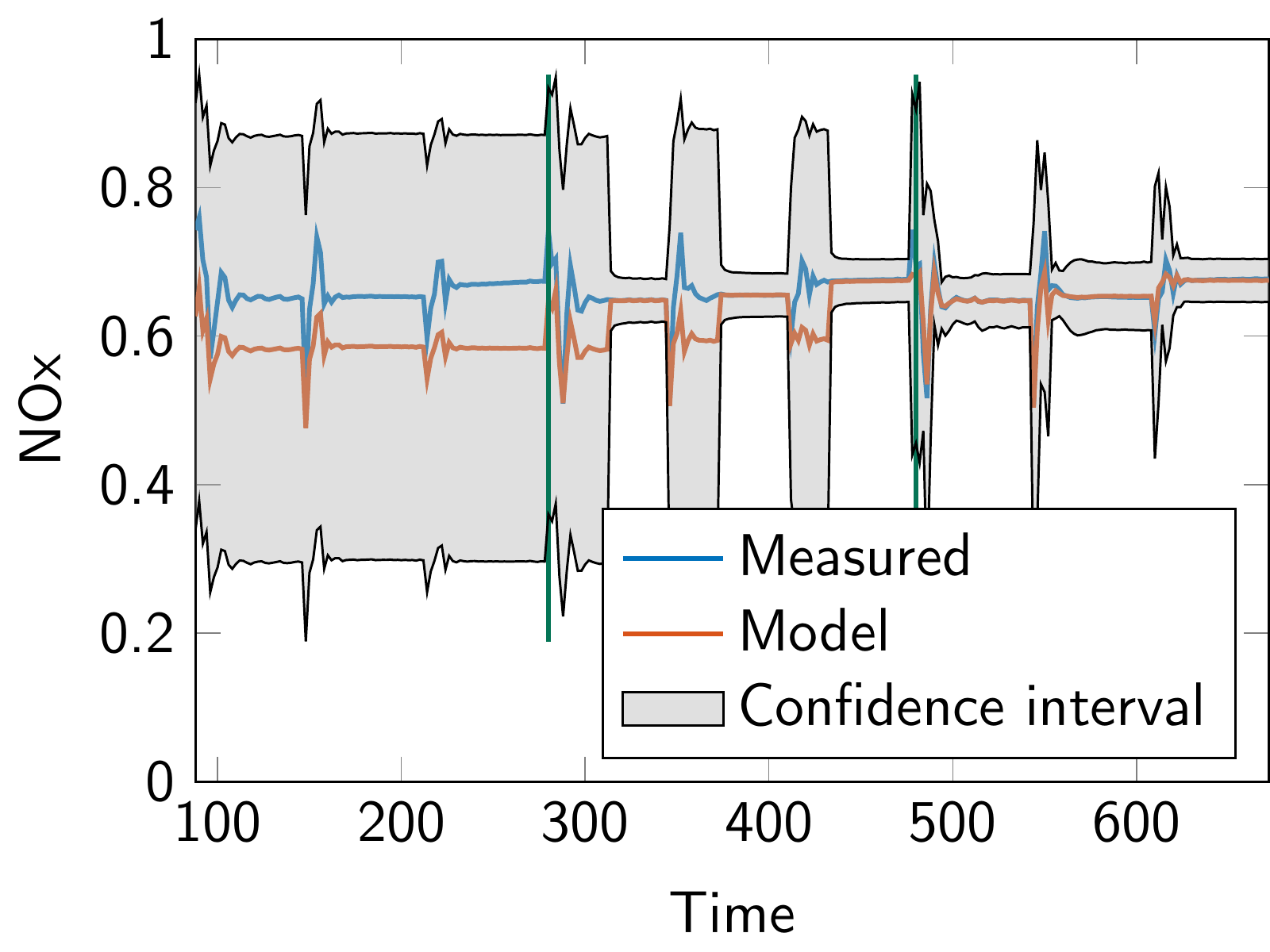
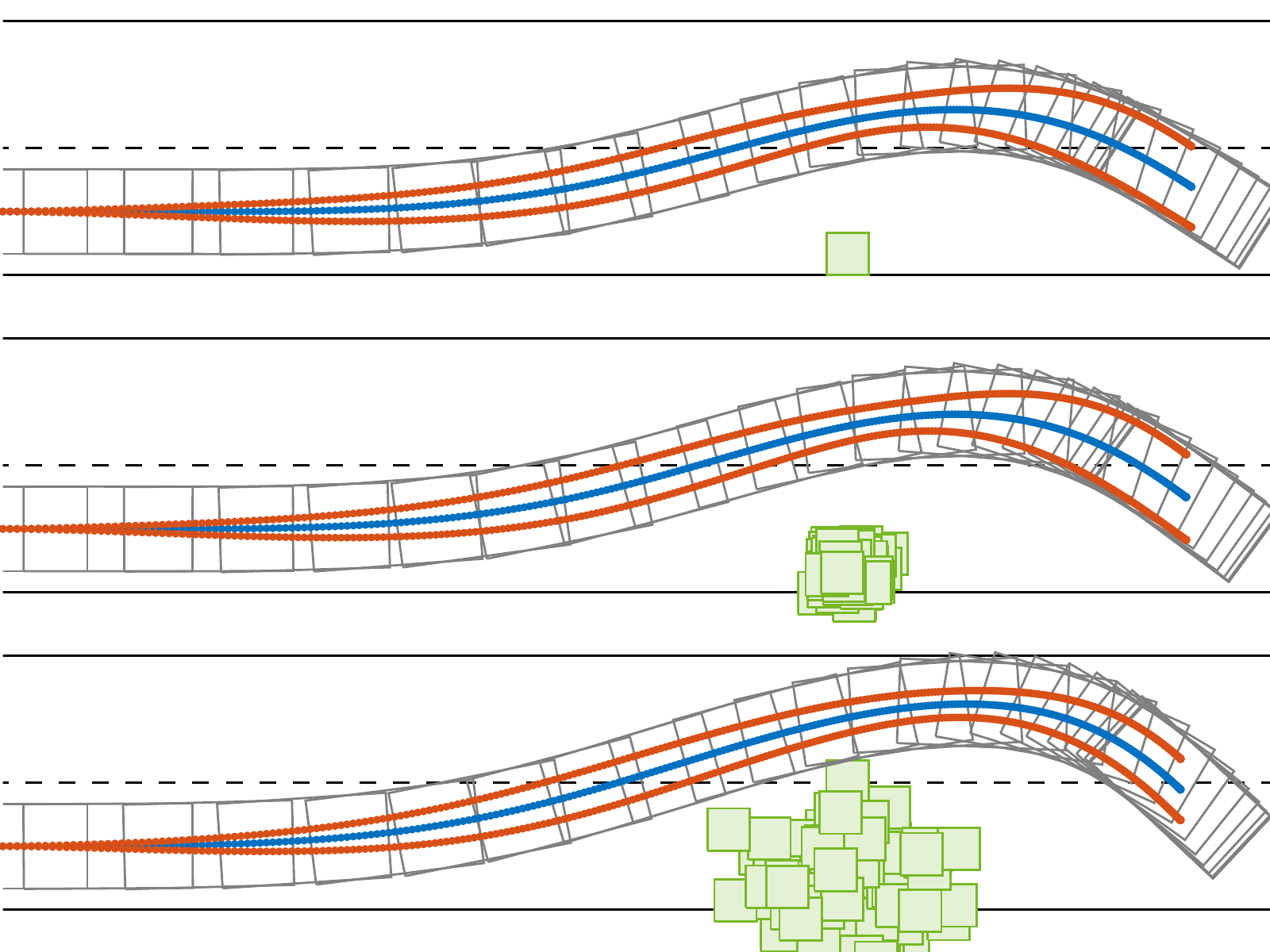
Another field of research and expertise is learning in optimization and control, for instance, reinforcement learning and Bayesian optimization. Reinforcement learning aims at obtaining an optimal control strategy from repeated interactions with the system. Formulating this task as an optimization problem shows the conceptual similarity to model predictive control, with the difference that reinforcement learning does not require model knowledge of the system. In a similar spirit, Bayesian optimization allows to solve complex optimization problem, in particular if the cost function or constraints are not analytically known or can only be evaluated by costly numerical simulations. Many technical tasks such as the optimization of production processes, an optimal product design or the search of optimal controller setpoints can be formulated as (partially) unknown optimization problem, illustrating the generality and importance of Bayesian optimization.
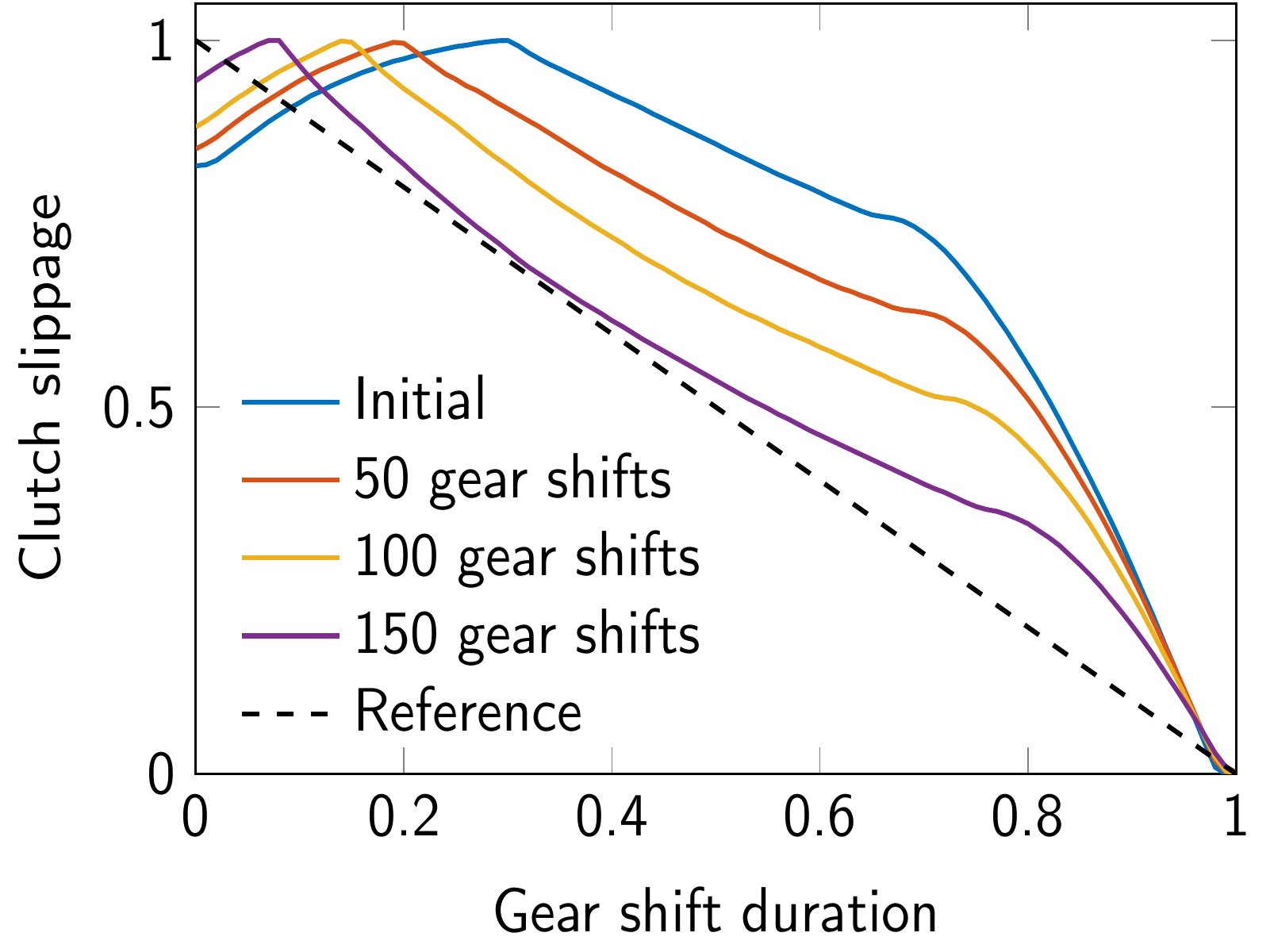
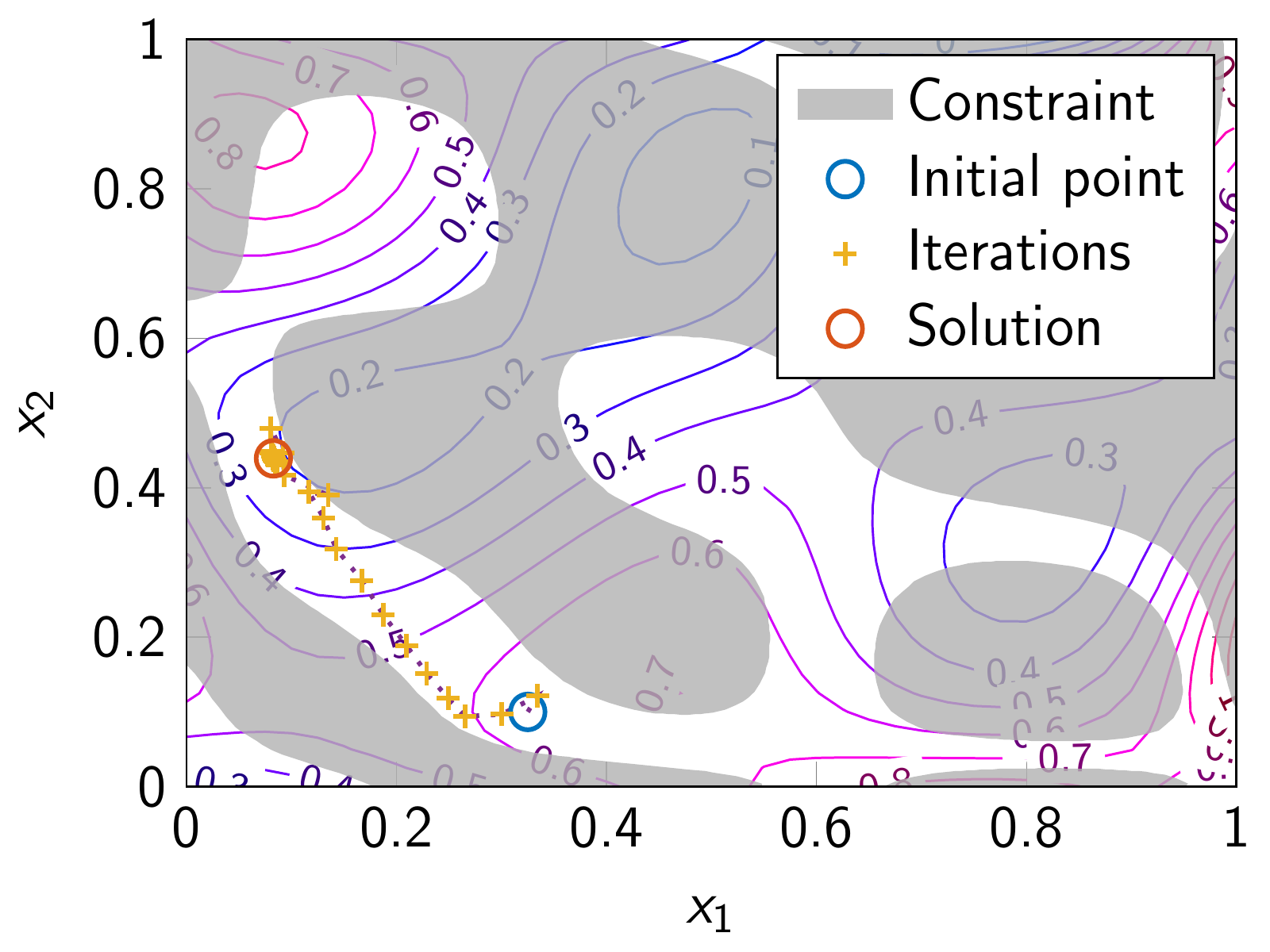
Related projects since 2021
Funding source: Industrie
Project leader:
Funding source: Deutsche Forschungsgemeinschaft (DFG)
Project leader:
Precise interactions as part of industrial manufacturing tasks are typically very complex to characterize and implement. One reason for this is the heterogeneity of the task-specific requirements for the motion and control behavior. A direct implementation of the task into a robot program therefore requires highly qualified specialists and is only profitable for large lot sizes. For a flexible applicability and easy (re-)configuration of the robot system, an approach to programming by kinesthetic…
Funding source: Bundesministerium für Wirtschaft und Klimaschutz (BMWK)
Project leader:
To achieve climate targets, CO2 emissions in the building sector have to be significantly reduced. However, the integration of renewable energy sources increases the complexity of building energy systems and thus the requirements for the operation strategy. Model-based and predictive controllers are necessary for efficient operation. However, due to the high complexity of the energy systems, the development, implementation, and commissioning are very complex leading to high costs, which is why…
Funding source: Bundesministerium für Bildung und Forschung (BMBF)
Project leader:
Funding source: Bundesministerium für Wirtschaft und Klimaschutz (BMWK)
Project leader:
Funding source: Industrie
Project leader:
Related publications
- Goller T, Brohm D, Völz A, Graichen K (2024). DMP-based path planning for model predictive interaction control. In European Control Conference (pp. 128-133).
- Goller T, Völz A, Graichen K (2024). A Programming by Demonstration Approach for Robotic Manipulation with Model Predictive Interaction Control. In 2024 IEEE Conference on Control Technology and Applications (CCTA) (pp. 799-804).
- Kißkalt J, Michalka A, Strohmeyer C, Horn M, Graichen K (2024). Fault detection in gauge-sensorized strain wave gears. In European Control Conference (pp. 26-33). [DOI].
- Pierer von Esch M, Landgraf D, Steffel M, Völz A, Graichen K (2024). Distributed Stochastic Optimal Control of Nonlinear Systems based on ADMM. IEEE Control Systems Letters, 8, 424-429. [DOI].
- Rabenstein G, Ullrich L, Graichen K (2024). Sampling for model predictive trajectory planning in autonomous driving using normalizing flows. In 5th IEEE Intelligent Vehicles Symposium (IEEE IV 2024) (pp. 2091-2096).
- Snobar F, Michalka A, Horn M, Strohmeyer C, Graichen K (2024). Sensitivity-based moving horizon estimation of road friction. In European Control Conference (pp. 718-724).
- Ullrich L, Buchholz M, Dietmayer K, Graichen K (2024). Expanding the Classical V-Model for the Development of Complex Systems Incorporating AI. IEEE Transactions on Intelligent Vehicles. [DOI].
- Ullrich L, McMaster A, Graichen K (2024). Transfer learning study of motion transformer based trajectory predictions. In 5th IEEE Intelligent Vehicles Symposium (IEEE IV 2024) (pp. 110-117).
- Wietzke T, Gall J, Graichen K (2024). Occupancy Prediction for Building Energy Systems with Latent Force Models. Energy and Buildings, pp. 113968. [DOI].
- Dio M, Demir O, Trachte A, Graichen K (2023). Safe active learning and probabilistic design of experiment for autonomous hydraulic excavators. In 2023 IEEE/RSJ International Conference on Intelligent Robots and Systems (IROS) (pp. 9685-9690).
- Kißkalt J, Michalka A, Strohmeyer C, Horn M, Graichen K (2023). Simulation chain for sensorized strain wave gears. In 27th International Conference on System Theory, Control and Computing (ICSTCC) (pp. 467 – 473). [DOI].
- Landgraf D, Völz A, Berkel F, Schmidt K, Specker T, Graichen K (2023). Probabilistic prediction methods for nonlinear systems with application to stochastic model predictive control. Annual Reviews in Control, 56, 100905. [DOI].
- Schumann M, Ebersberger S, Graichen K (2023). Online learning and adaptation of nonlinear thermal networks for power inverters. In 49th Annual Conference of the IEEE Industrial Electronics Society (IECON 2023).
- Schumann M, Ebersberger S, Graichen K (2023). Improved nonlinear estimation in thermal networks using machine learning. In IEEE International Conference on Mechatronics (ICM 2023). [DOI].
- Snobar F, Michalka A, Horn M, Strohmeyer C, Graichen K (2023). Rack force estimation from standstill to high speeds by hybrid model design and blending. In IEEE International Conference on Mechatronics (ICM 2023). [DOI].
- Spenger P, Graichen K (2023). Performance prediction of NMPC algorithms with incomplete optimization. In 22nd IFAC World Congress (pp. 7456-7461).
- Ullrich L, Völz A, Graichen K (2023). Robust meta-learning of vehicle yaw rate dynamics via conditional neural processes. In 62nd IEEE Conference on Decision and Control (CDC).
- Bergmann D, Harder K, Niemeyer J, Graichen K (2022). Nonlinear MPC of a Heavy-Duty Diesel Engine With Learning Gaussian Process Regression. IEEE Transactions on Control Systems Technology, 30(1), 113-129. [DOI].
- Goller T, Gold T, Völz A, Graichen K (2022). Model predictive interaction control based on a path-following formulation. In Proceedings IEEE International Conference on Mechatronics and Automation (ICMA) (pp. 551-556). [DOI].
- Landgraf D, Völz A, Kontes G, Graichen K, Mutschler C (2022). Hierarchical learning for model predictive collision avoidance. In 10th Vienna International Conference on Mathematical Modelling (MATHMOD 22) (pp. 355-360). [DOI].
- Rabenstein G, Demir O, Trachte A, Graichen K (2022). Data-driven feed-forward control of hydraulic cylinders using Gaussian process regression for excavator assistance functions. In 6th IEEE Conference on Control Technology and Applications (CCTA) (pp. 962-969). [DOI].
- Schumann M, Ebersberger S, Graichen K (2022). Dynamic and stationary state estimation of fluid cooled three-phase inverters. In 26th IEEE International Symposium on Power Electronics, Electrical Drives Automation and Motion (SPEEDAM 2022). [DOI].
- Stecher J, Kiltz L, Graichen K (2022). Semi-infinite programming using Gaussian process regression for robust design optimization. In Proceedings European Control Conference (pp. 52-59). [DOI].
- Bergmann D, Graichen K (2020). Safe Bayesian Optimization under Unknown Constraints. In 59th IEEE Conference on Decision and Control, CDC 2020 (pp. 3592-3597). [DOI].
- Geiselhart R, Bergmann D, Niemeyer J, Remele J, Graichen K (2020). Hierarchical Predictive Control of a Combined Engine/Selective Catalytic Reduction System with Limited Model Knowledge. SAE International Journal of Engines, 13(2). [DOI].
- Geiselhart R, Bergmann D, Niemeyer J, Remele J, Graichen K (2020). Hierarchical predictive control of a combined engine/SCR system with limited model knowledge. SAE International Journal of Engines, 13(2). [DOI].
- Mesmer F, Szabo T, Graichen K (2020). Learning feedforward control of a hydraulic clutch actuation path based on policy gradients. In 59th IEEE Conference on Decision and Control (CDC 2020). [DOI].
- Bergmann D, Geiselhart R, Graichen K (2019). Modelling and control of a heavy-duty Diesel engine gas path with Gaussian process regression. In Proc. European Control Conference (ECC 2019) (pp. 1207-1213). Naples (Italy). [DOI].
- Bergmann D, Graichen K (2019). Gaußprozessregression zur Modellierung zeitvarianter Systeme. At-Automatisierungstechnik, 67(8), 637-647. [DOI].
- Mesmer F, Szabo T, Graichen K (2019). Learning methods for the feedforward control of a hydraulic clutch actuation path. In Proc. IEEE/ASME International Conference on Advanced Intelligent Mechatronics (AIM 2019) (pp. 733-738). Hong Kong (China). [DOI].