Energy Solutions
The energy-efficient operation of technical systems is of increasing importance in view of global warming and the transition to renewable energies. Control systems engineering has a high potential to contribute to the efficient use of energy, for example, regarding energy consumption of buildings, energy conversion and storage, as well as power grids. Our research in terms of optimization and learning-based control methods forms the ideal basis to develop sustainable solutions in energy-related applications.
The efficient and sustainable use of energy is one of the grand challenges today. Control engineering is a key technology to accomplish this goal by providing tailored automation solutions. For instance, heating, ventilation and air conditioning (HVAC) systems in residential and non-residential buildings belong to the largest energy consumers. Advanced control schemes can help to efficiently control HVAC systems while learning the building characteristics like thermal dynamics or room occupancy patterns and considering stochastic weather forecasts to reduce the energy consumption in a predictive manner.
Another example are electrical grids that connect energy sources (power plants, wind turbines, etc.) and energy sinks (e.g. factories, households). On the lower level, each involved component, e.g. drives and inverters, can be designed to reduce undesired power dissipation. On the higher level, the energy distribution itself can be optimized using, for instance, distributed model predictive control for smart grids.
Despite the current prevalence of batteries as mobile energy storages, hydrogen-based fuel cells are a promising alternative for applications with high energy demand as, for example, trains or ships. One option to solve the problem of save hydrogen storage is the use of liquid organic hydrogen carriers (LOHC), either as storage/transport medium or for direct fuel cell usage. The de-/hydrogenation of LOHC is a complex process that offers high potential for improvement by modern control methods.
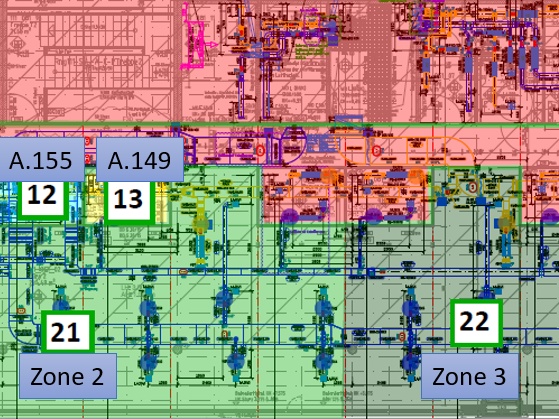
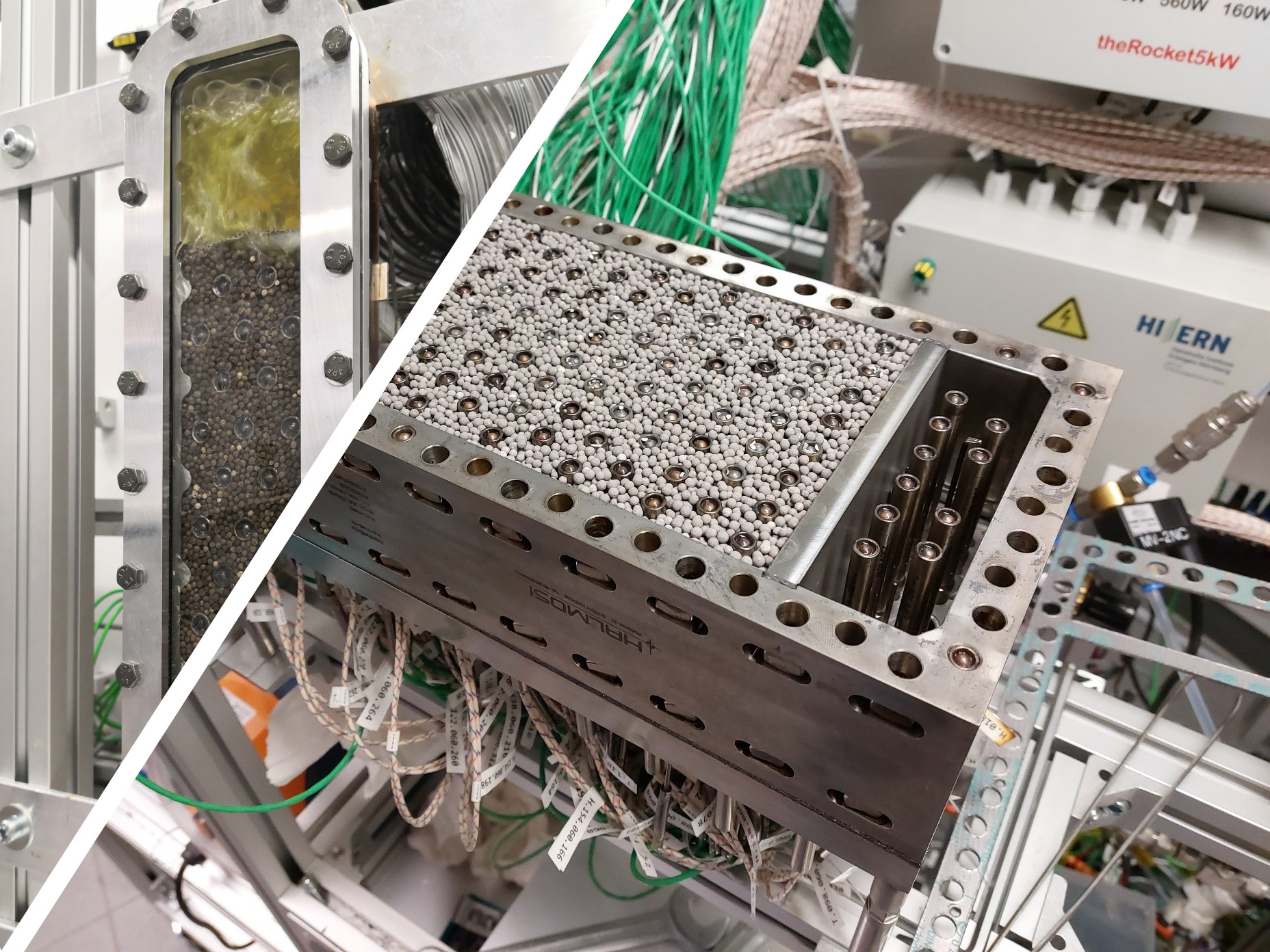
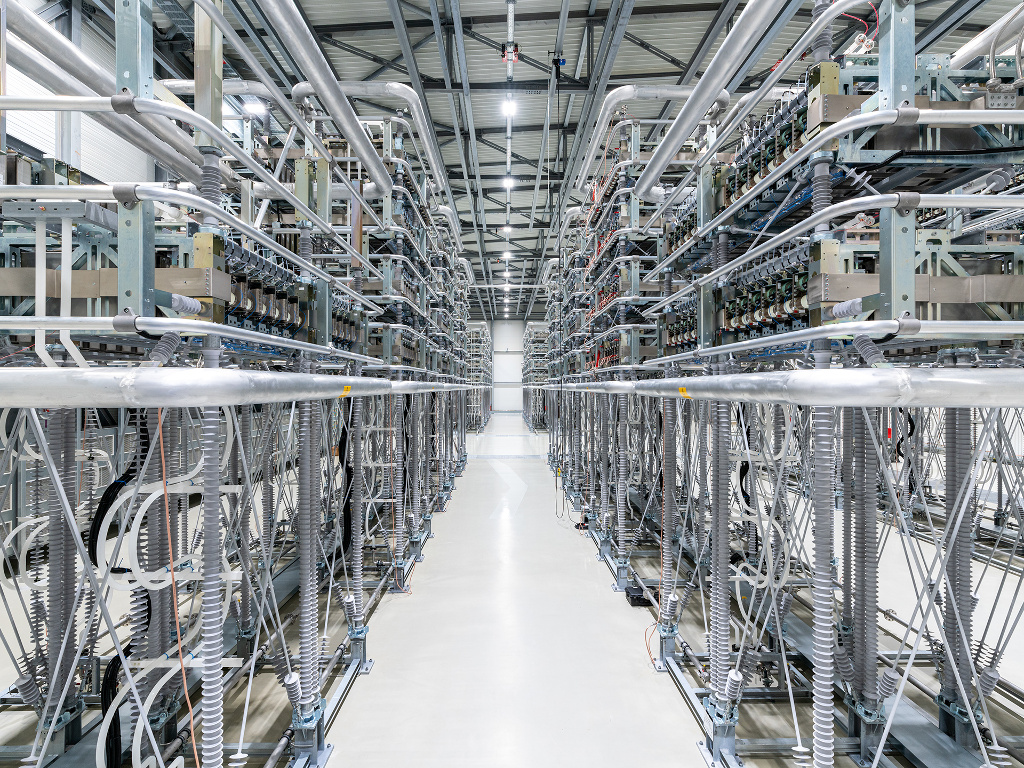
Related projects since 2021
Funding source: Bundesministerium für Wirtschaft und Klimaschutz (BMWK)
Project leader:
To achieve climate targets, CO2 emissions in the building sector have to be significantly reduced. However, the integration of renewable energy sources increases the complexity of building energy systems and thus the requirements for the operation strategy. Model-based and predictive controllers are necessary for efficient operation. However, due to the high complexity of the energy systems, the development, implementation, and commissioning are very complex leading to high costs, which is why…
Related publications
- Landgraf D, Wietzke T, Graichen K (2025). Stochastic model predictive control with switched latent force models. In European Control Conference.
- Wietzke T, Graichen K (2025). Physics-informed sparse Gaussian processes for model predictive control in building energy systems. In 11th Vienna International Conference on Mathematical Modelling (MATHMOD 25).
- Wietzke T, Landgraf D, Graichen K (2025). Application of stochastic model predictive control for building energy systems using latent force models. At-Automatisierungstechnik, (accepted).
- Verhoolen A, Geißelbrecht M, Kadar J, Preuster P, Wasserscheid P, Graichen K (2024). Bayesian optimization of operating points of a continuous perhydro-dibenzyltoluene dehydrogenation reactor. International Journal of Energy Research. [DOI].
- Wietzke T, Gall J, Graichen K (2024). Occupancy Prediction for Building Energy Systems with Latent Force Models. Energy and Buildings, pp. 113968. [DOI].
- Schumann M, Ebersberger S, Graichen K (2023). Online learning and adaptation of nonlinear thermal networks for power inverters. In 49th Annual Conference of the IEEE Industrial Electronics Society (IECON 2023).
- Schumann M, Ebersberger S, Graichen K (2023). Improved nonlinear estimation in thermal networks using machine learning. In IEEE International Conference on Mechatronics (ICM 2023). [DOI].
- Huber H, Burk D, Graichen K (2022). Comparison of sensitivity-based and ADMM-based DMPC applied to building automation. In 6th IEEE Conference on Control Technology and Applications (CCTA) (pp. 546-553). [DOI].
- Kowalewski J, Lorenz A, Lomakin A, Alvarez R, Graichen K (2022). Circulating current control and energy balancing of a modular multilevel converter using model predictive control for HVDC applications. In 48th Annual Conference of the IEEE Industrial Electronics Society (IECON 2022). [DOI].
- Schumann M, Ebersberger S, Graichen K (2022). Dynamic and stationary state estimation of fluid cooled three-phase inverters. In 26th IEEE International Symposium on Power Electronics, Electrical Drives Automation and Motion (SPEEDAM 2022). [DOI].
- Huber H, Graichen K (2021). A sensitivity-based distributed model predictive control algorithm for nonlinear continuous-time systems. In 5th IEEE Conference on Control Technology and Applications (CCTA) (pp. (accepted)). [DOI].